The Transformational Impact of Machine Learning Data Labeling in Business
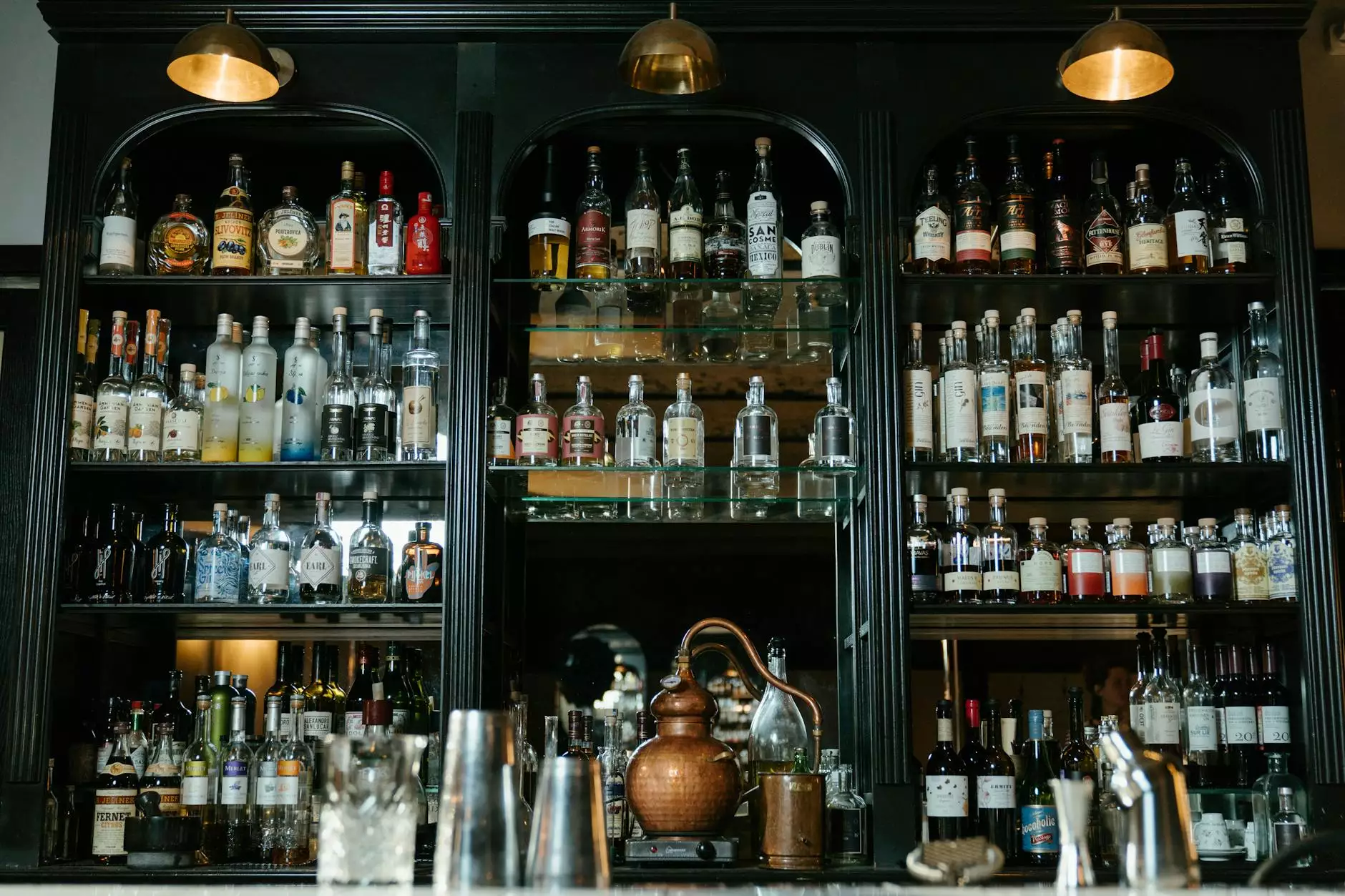
In today's technology-driven world, the power of machine learning data labeling is reshaping how businesses operate across various sectors. Industries such as home services and locksmiths are no exception. This comprehensive article delves into the significance of data labeling in machine learning, its applications in business, and how it can serve as a competitive advantage.
Understanding Machine Learning and Data Labeling
Machine learning is a subset of artificial intelligence that enables systems to learn from data, improve their performance, and make decisions without being explicitly programmed. For machine learning algorithms to function accurately, they require high-quality, labeled data. This is where data labeling comes into play.
What Is Data Labeling?
Data labeling is the process of annotating or tagging data to provide context to machine learning algorithms. This process can involve:
- Image labeling: Tagging objects within images for computer vision tasks.
- Text labeling: Classifying snippets of text to aid natural language processing.
- Audio labeling: Identifying voice commands or sounds for speech recognition systems.
The Importance of Data Labeling in Machine Learning
Accurate data labeling is crucial in ensuring the success of machine learning models. When the data is well-labeled, the model can learn more efficiently and accurately, leading to better predictions and insights.
Benefits of Accurate Data Labeling
Here are some of the key benefits of investing in machine learning data labeling:
- Improved Accuracy: Well-labeled data minimizes errors and enhances the overall accuracy of the models.
- Enhanced Model Performance: High-quality training data leads to better performance in real-world applications.
- Scalability: Properly labeled datasets allow businesses to scale their models efficiently.
- Time Efficiency: A well-labeled dataset reduces the amount of time spent refining models.
Applications of Machine Learning Data Labeling in Businesses
Businesses across various sectors leverage machine learning data labeling to optimize operations, enhance customer experiences, and improve decision-making. Here are some prominent applications:
1. Home Services
Within the home services sector, data labeling plays a vital role in service optimization, customer relationship management, and predictive maintenance.
Service Optimization
For companies involved in home repairs and maintenance, data labeling allows for analyzing customer feedback and service requests. By labeling customer data, businesses can identify common problems and tailor their services accordingly. For instance:
- Labeling types of repair requests allows for resource allocation.
- Identifying peak seasons for specific services helps in planning workforce management.
Predictive Maintenance
Using labeled historical data from service jobs, companies can develop predictive models that indicate when maintenance should be performed on home appliances or systems. This proactive approach minimizes service interruptions and enhances customer satisfaction.
2. Locksmith Services
In the keys and locksmiths industry, machine learning can be used to enhance security systems and improve customer service.
Security Analytics
Locksmith businesses can leverage labeled data from security incidents to develop machine learning models that predict potential break-ins or security breaches. For instance,:
- Labeling types of security incidents can help identify patterns.
- Analyzing geographical data can highlight the most vulnerable neighborhoods.
Customer Service Improvement
By utilizing customer interaction data and labeling it appropriately, locksmith companies can train chatbots or customer service agents to respond more effectively. This leads to:
- Faster response times.
- Improved customer satisfaction rates.
How to Implement Efficient Data Labeling in Your Business
To maximize the benefits of machine learning data labeling, businesses must adopt effective strategies. Here are some steps to consider:
1. Define Clear Objectives
Before beginning the data labeling process, it's essential to define clear objectives. Understand what you want to achieve with your machine learning models and how labeled data can help. This could involve identifying:
- Specific problems you want to solve.
- Target outcomes for your models.
2. Choose the Right Data Labeling Tools
Invest in reliable data labeling tools that facilitate easy and accurate labeling. Some popular options include:
- Labelbox: A versatile platform for image and video annotation.
- SuperAnnotate: Excellent for handling large datasets efficiently.
- Amazon SageMaker: Provides built-in labeling by leveraging human annotators.
3. Invest in Skilled Labelers
Your labeling efforts will only be as good as the individuals performing the labeling. Invest in training or hire skilled annotators familiar with the specifics of your business. Consider:
- Offering training sessions to help labelers better understand your requirements.
- Evaluating the quality of labeled data regularly to ensure consistency and accuracy.
4. Continuously Monitor and Update Your Datasets
Machine learning is a dynamic field. As new data emerges, your datasets need to be updated. Regularly monitor the performance of your models and iterate your labeling processes to maintain high accuracy levels.
Conclusion: The Future of Business with Machine Learning Data Labeling
As businesses increasingly embrace technology, the role of machine learning data labeling will only become more significant. For home services and locksmiths, effectively leveraging labeled data will provide a pathway to greater innovation, efficiency, and customer satisfaction.
By understanding the power of data labeling and implementing it effectively, businesses can not only keep pace with industry changes but also establish themselves as leaders in their respective fields. The future holds immense potential for those who harness the capabilities of machine learning alongside well-labeled data.